Temperature and vegetation variation within a rapidly growing town
As a first-year MSc student at the University of Toronto Mississauga, I wrote a paper for a planning course where I integrated remote sensing data with planning documents to understand environmental change in the rapidly growing Town of Milton, Ontario. In this blog post, I will summarize the paper including my methods and findings. However, I will not touch on the integration with planning documents.
Climate change is creating a shift in climate patterns, caused by natural systems and human activity (Fawzy et al., 2020). The Earth has been warming for decades, suggesting that global temperatures will increase between 0.8 and 1.5 °C between 2030 and 2052 (Field et al., 2012; Masson-Delmotte et al., 2018). One of the contributions caused by human activity is the change in land use/land cover (LULC), as it results in lost agricultural land and forest area, leading to an increase in barren area and impermeable surfaces (Buyadi et al., 2013; Fall et al., 2010; Hussain et al., 2014; Kant et al., 2009; Kumar et al., 2012). Cities are losing greenery due to rapid urbanization from LULC change, contributing to the urban heat island (UHI) effect (Buyadi et al., 2013). The UHI effect explains the condition where urban areas are hotter than their surrounding rural areas, due to the increase of heat absorbing surfaces from human infrastructure (Buyadi et al., 2013; Guo et al., 2012; Weng, 2001; Xiao & Weng, 2007; Shahmohamadi et al., 2011). It’s important to analyze historic and current LULC to understand and manage the UHI effect (Abd El-Kawy et al., 2011; Buyadi et al., 2013; Das, 209; Pelorosso et al., 2009).
Land surface temperature (LST) data from remote sensing satellite imagery allows us to understand environmental change associated with land development and UHI effects (Kant et al., 2009; Anderson et al., 2008; Arnfield, 2003). Luckily, with long-term satellite data, we can detect, quantify, and monitor change (Chen et al., 2005; Lu et al., 2004). Many studies have determined that LST and LULC are strongly related to the UHI effect (Kant et al., 2009).
In this paper, I investigated remote sensing data to understand environmental change within the Town of Milton, Ontario, an area undergoing rapid landscape change. I wanted to discover if the relationship between LST and the Normalized Difference Vegetation Index (NDVI) can determine if a decrease in vegetation increases urban heat exposure.
North America has experienced extreme weather conditions such as rising temperatures, droughts, and wildfires, resulting in morbidity, mortality, respiratory health issues, and diseases (United Nations, n.d.). Drought is the main concern for agriculture because of the loss of moisture in the ground (Cook et al., 2018). The Province of Ontario will see 20-30% of plant species at high risk of extinction because of the increase in global temperatures (Office of Auditor General of Ontario, n.d.). However, Ontario has been implementing nature-based solutions to help mitigate climate change, such as the Ontario Greenbelt Plan (2005), aiming to protect 2 million acres of land from urban sprawl (Green Belt, n.d.).
The Town of Milton is a compact suburban community in the Greater Toronto Area (GTA), within which the Niagara Escarpment creates distinct urban and rural areas (Statistics Canada, n.d.; Town of Milton, 2008). This study investigates the correlation between temperature and vegetation to understand the possible risks of rapid urban expansion.
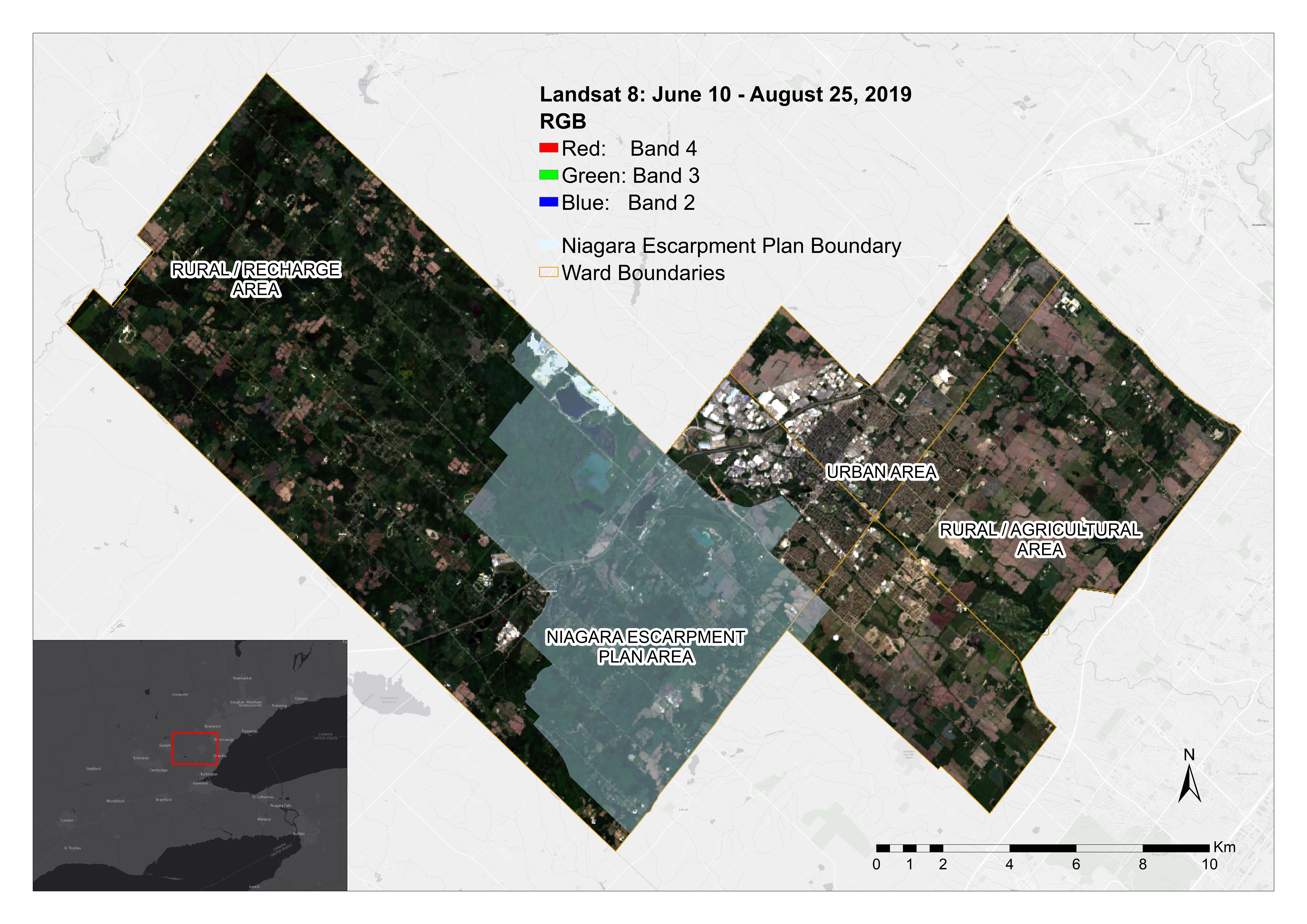
Remote sensing was used to extract LST and NDVI data for Milton using Google Earth Engine (GEE) obtained from USGS (Gorelick et al., 2017; USGS, n.d.). The images were obtained for the summer months in 10-year increments from 1989, 1999, 2009, and 2019, using Landsat 5-TM (1989, 1999, and 2009) and Landsat 8-OLI/TIRS (2019). The best and most available data from June 10th to August 25th were selected. The LST images were converted from Kelvin to Celsius in ArcGIS Pro. The NDVI images were calculated using the red and near-infrared bands, also in ArcGIS Pro. I also obtained historic ground-level meteorological data from Toronto Pearson Airport to validate the LST data (Government of Canada, n.d.).
Average Mean Temperature (°C) | Average Maximum Temperature (°C) | |
1989 | 20.05 | 25.73 |
1999 | 21.94 | 27.13 |
2009 | 19.93 | 24.86 |
2019 | 21.65 | 26.68 |
Starting with the LST results, the maximum temperatures from all four years are between 38 and 54 °C, and the minimum temperatures are between 5 and 25 °C. Overall, the coolest temperatures are in the rural area (west) and the warmest temperatures are in the urban area (east). However, 1999 has hot areas in the rural area (west), suggesting a hot year. The weather station data corroborates this finding. 2009 looks like a cold year, as seen through the LST results and the weather station data. In 2019, the size of the hot area expands within the urban area (east).

The LST and weather station data show a similar pattern. Temperatures increased from 1989 to 1999, decreased in 2009, and increased in 2019. There is a 5 to 7 °C variation between the two datasets. It is important to note that the weather station data is from 30 kilometres away from the center of the Town of Milton.

Moving on to the NDVI results, the maximum NDVI for all four years is between 0.88 and 0.91, and the minimum is between -0.16 and 0.1. Overall, higher NDVI is found in the rural area (west) and lower NDVI in the urban area (east). We can see this trend in 1989 (Figure 2). Within each year, we can see a decrease in NDVI occurring in the urban area (east). However, we can see that the Niagara Escarpment has consistent dense vegetation (Figure 2).
200 random ground points were used to extract LST and NDVI to create linear regression models to investigate their relationship (Figure 4). Land type was determined by using unsupervised classification and was subsequently used for analysis. The linear regression models showed a significant negative relationship between LST and NDVI. From the overall results, for every 0.1 decrease in NDVI, LST increases about 1.17 °C. Visually, vegetation points see higher NDVI values and lower LST values, while non-vegetation points, such as urban, residential, and bare soil areas, see lower NDVI values and higher LST values.
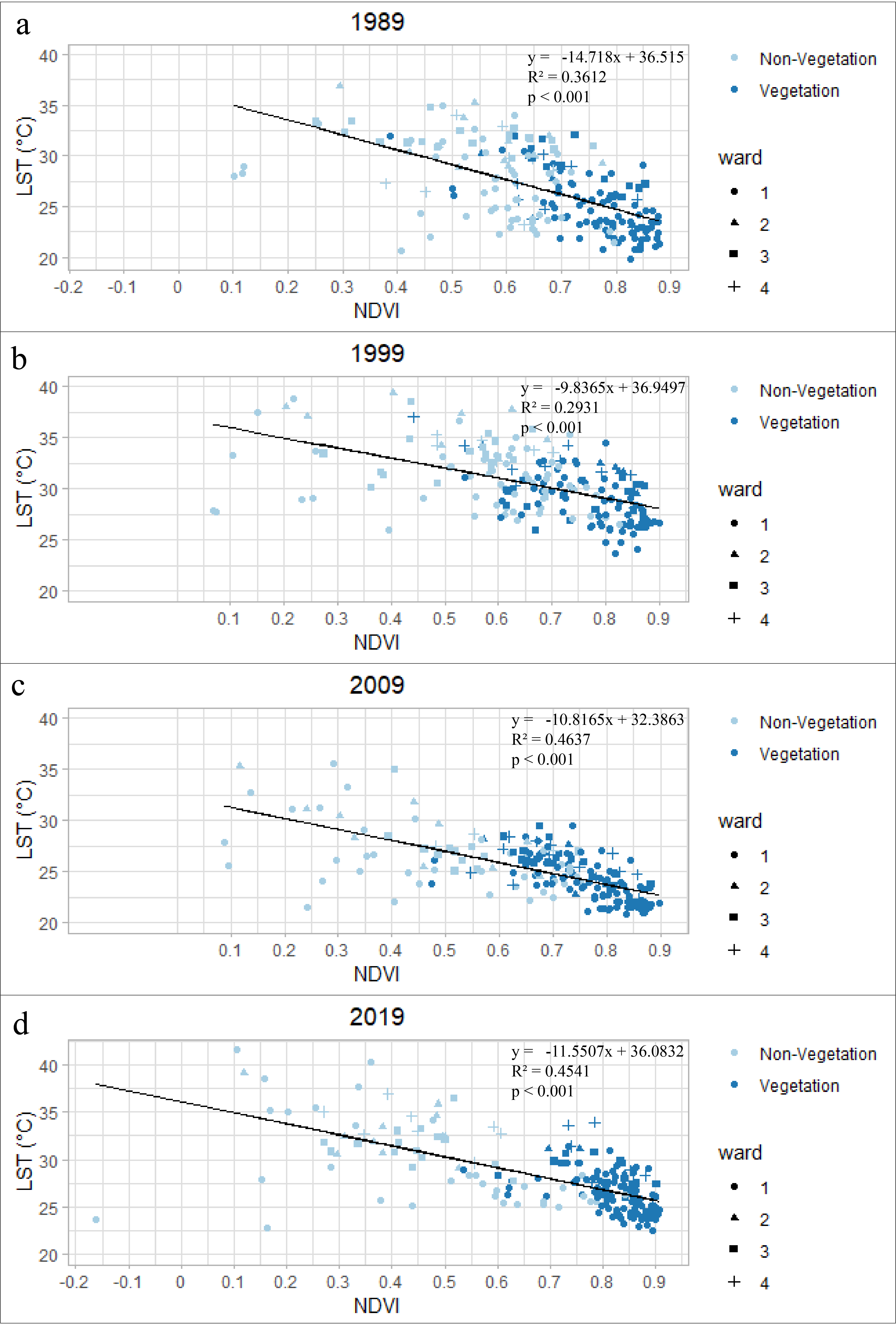
Overall, the remote sensing results recognize a relationship between temperature and vegetation density. From 1989 to 2019, we saw a decrease in dense vegetation in the urban area (east). These results can be combined with known development information. Between 1996 and 2016, Milton built 23,700 housing units to accommodate its growing population (Malone Given Parsons Ltd., 2017). The Greenbelt Plan (2005) protects the Niagara Escarpment and its agricultural areas (Green Belt, n.d.). Using the maps (Figure 2), we can see a visual relationship between LST and NDVI as areas with high temperatures are associated with low NDVI, and vice versa.
This study used remote sensing data to understand how urban expansion is linked to increasing temperatures. I hope this information leads to a discussion on how to implement green mitigation strategies in urban areas to mitigate the UHI effect.
– Scarlett Rakowska
References
Abd El-Kawy, O. R., Rød, J. K., Ismail, H. A. & Suliman, A. S. Land use and land cover change detection in the western Nile delta of Egypt using remote sensing data. Appl. Geogr. 31, (2011).
Anderson, M. C. et al. A thermal-based remote sensing technique for routine mapping of land-surface carbon, water and energy fluxes from field to regional scales. Remote Sens. Environ. 112, 4227–4241 (2008).
Arnfield, A. J. Two decades of urban climate research: A review of turbulence, exchanges of energy and water, and the urban heat island. Int. J. Climatol. 23, (2003).
Buyadi, S. N. A., Mohd, W. M. N. W. & Misni, A. Impact of Land Use Changes on the Surface Temperature Distribution of Area Surrounding the National Botanic Garden, Shah Alam. Procedia – Soc. Behav. Sci. 101, (2013).
Chen, X., Vierling, L. & Deering, D. A simple and effective radiometric correction method to improve landscape change detection across sensors and across time. Remote Sens. Environ. 98, (2005).
Cook, B. I., Mankin, J. S. & Anchukaitis, K. J. Climate Change and Drought: From Past to Future. Current Climate Change Reports vol. 4 (2018).
Das, T. Land Use / Land Cover Change Detection : an Object Oriented Approach, Münster, Germany Thesis. Thesis (2009).
Fall, S. et al. Impacts of land use land cover on temperature trends over the continental United States: Assessment using the North American Regional Reanalysis. Int. J. Climatol. 30, (2010).
Fawzy, S., Osman, A. I., Doran, J. & Rooney, D. W. Strategies for mitigation of climate change: a review. Environmental Chemistry Letters vol. 18 (2020).
Field, C. B. et al. Managing the risks of extreme events and disasters to advance climate change adaptation: Special report of the intergovernmental panel on climate change. Managing the Risks of Extreme Events and Disasters to Advance Climate Change Adaptation: Special Report of the Intergovernmental Panel on Climate Change vol. 9781107025066 (2012).
Gorelick, Noel and Hancher, Matt and Dixon, Mike and Ilyushchenko, Simon and Thau, David and Moore, R. Google Earth Engine: Planetary-scale geospatial analysis for everyone. (2017) doi:10.1016/j.rse.2017.06.031.
Government of Canada. Historical Data. https://climate.weather.gc.ca/historical_data/search_historic_data_e.html.
Green Belt. Green Belt. https://www.greenbelt.ca/.
Guo, Z., Wang, S. D., Cheng, M. M. & Shu, Y. Assess the effect of different degrees of urbanization on land surface temperature using remote sensing images. Procedia Environ. Sci. 13, (2012).
Hussain, A., Bhalla, P. & Palria, S. Remote sensing based analysis of the role of land use/land cover on surface temperature and temporal changes in temperature a case study of Ajmer District, Rajasthan. in International Archives of the Photogrammetry, Remote Sensing and Spatial Information Sciences – ISPRS Archives vol. 40 (2014).
Kant, Y., Bharath, B. D., Mallick, J., Atzberger, C. & Kerle, N. Satellite-based analysis of the role of land use/land cover and vegetation density on surface temperature regime of Delhi, india. J. Indian Soc. Remote Sens. 37, (2009).
Kumar, K. S., Bhaskar, P. U. & Padmakumari, K. Estimation of Land Surface Temperature To Study Urban Heat Island Effect Using Landsat Etm+ Image. Int. J. Eng. Sci. Technol. 4, (2012).
Lu, D., Mausel, P., Brondízio, E. & Moran, E. Change detection techniques. Int. J. Remote Sens. 25, (2004).
Malone Given Parsons Ltd. Land Base Analysis. https://www.milton.ca/en/business-and-development/resources/South-East-Milton-Urban-Expansion-Land-Base-Analysis.pdf (2017).
Masson-Delmotte, V., P. Zhai, H.-O. Pörtner, D. Roberts, J. Skea, P. R. S., A. Pirani, W. Moufouma-Okia, C. Péan, R. Pidcock, S. Connors, J.B.R. Matthews, Y. Chen, X. Zhou, M.I. Gomis, E. Lonnoy, T. M. & M. Tignor, and T. W. (eds. . IPCC, 2018: Global Warming of 1.5°C.An IPCC Special Report on the impacts of global warming of 1.5°C above pre-industrial levels and related global greenhouse gas emission pathways, in the context of strengthening the global response to the threat of clim.
Office of Auditor General of Ontario. Chapter 3: Climate Change: Ontario’s Plan to Reduce Greenhouse Gas Emissions. https://www.auditor.on.ca/en/content/annualreports/arreports/en19/v2_300en19.pdf.
Pelorosso, R., Leone, A. & Boccia, L. Land cover and land use change in the Italian central Apennines: A comparison of assessment methods. Appl. Geogr. 29, (2009).
Shahmohamadi, P., Che-Ani, A. I., Maulud, K. N. A., Tawil, N. M. & Abdullah, N. A. G. The Impact of Anthropogenic Heat on Formation of Urban Heat Island and Energy Consumption Balance. Urban Stud. Res. 2011, (2011).
Statistics Canada. Canada’s fastest growing and decreasing municipalities from 2016 to 2021. https://www12.statcan.gc.ca/census-recensement/2021/as-sa/98-200-x/2021001/98-200-x2021001-eng.cfm.
Town of Milton. Town of Milton Official Plan. (2008).
United Nations. Taking Climate Action. https://www.unep.org/regions/north-america/regional-initiatives/taking-climate-action#:~:text=Over the years%2C the North,be more difficult and costly.
(USGS), the U. S. G. S. Landsat Collection 2 Level-2 Science Products.
Weng, Q. A remote sensing?GIS evaluation of urban expansion and its impact on surface temperature in the Zhujiang Delta, China. Int. J. Remote Sens. 22, (2001).
Xiao, H. & Weng, Q. The impact of land use and land cover changes on land surface temperature in a karst area of China. J. Environ. Manage. 85, (2007).